Data Unlocked:
Part I: Fundamental concepts & principles
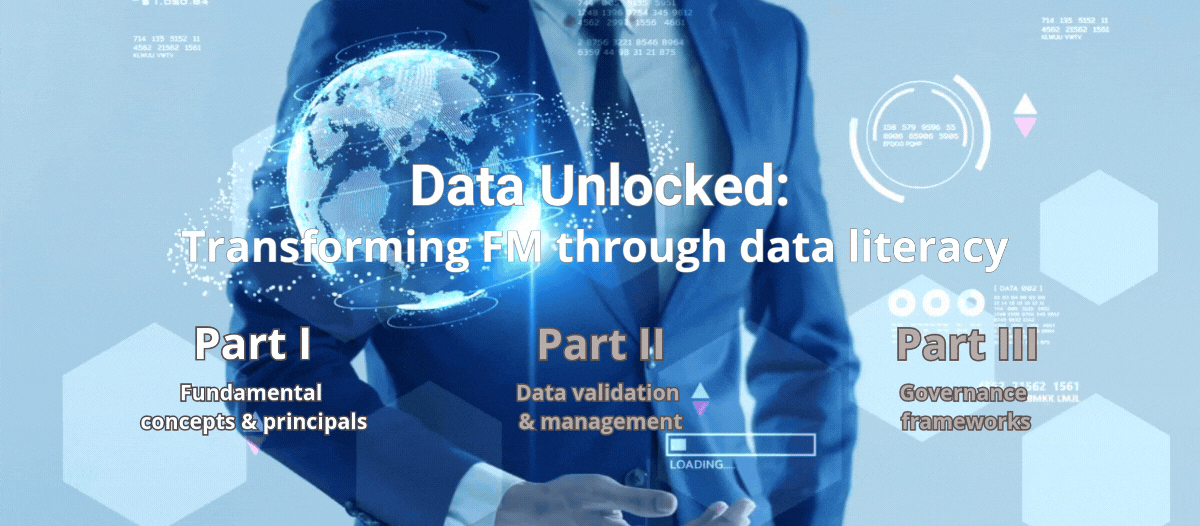
Editor’s note: This is the first article in a three-part series discussing the fundamental concepts of data literacy, validation and governance in FM.
Facility management is undergoing a profound transformation, driven by the increasing availability of data. But data without the skills to use it is like having a map but no idea how to read it.
Why data literacy matters in FM
Imagine managing a building without any blueprints, not knowing where pipes, wiring or crucial components are located. Similarly, managing facilities today without a solid understanding of data is like running blindfolded. In FM, data is at the core of almost every function — whether it is energy optimization, predictive maintenance, occupancy analysis or asset management. Data literacy empowers FM professionals to make informed decisions that improve efficiency, enhance sustainability and elevate occupant satisfaction. Just as a well-designed HVAC system keeps air flowing smoothly through a building, data literacy keeps relevant information flowing through decision-making processes, reducing costly errors and enhancing proactive management.
Moreover, data literacy plays a vital role in bridging communication gaps across teams. Whether it is collaborating with financial departments to justify operational budgets or working with sustainability teams to meet energy efficiency goals, a solid grasp of data helps FM professionals articulate insights and drive evidence-based conversations. In a world where decisions are increasingly data-driven, the ability to understand, interpret and use data effectively is what separates a good FM from a great one.
Key concepts in data literacy for FM professionals
Data literacy defined
Data literacy refers to the ability to read, work with, analyze and communicate with data. Think of it like learning a new language: just as one must know the grammar and vocabulary of a language to effectively communicate, data literacy requires an understanding of data concepts and how to apply them. The good news is that, like learning any new language, mastering data literacy starts with small steps: basic definitions, structures and interpreting meaning. For FM professionals, this means being able to interpret energy consumption charts, extract valuable information from maintenance logs and communicate findings to stakeholders who may not be as data savvy.
Types of data
Data can come in many different types and formats. Imagine data types as different tools in a toolbox, each designed for a specific purpose. FMs work with two primary categories: structured data and unstructured data. Structured data is like a neatly organized spreadsheet; think of asset inventories, maintenance schedules or budget reports. Everything fits into nice, defined rows and columns. In contrast, unstructured data is like a junk drawer. It contains important information, but it does not conform to a specific format. Examples include maintenance logs written as text, images from building inspections or sensor data that have not been standardized.
Data in FM comes in many forms, each with unique applications. Beyond structured data (like asset inventories) and unstructured data (like free-text maintenance logs), semi-structured data plays a critical role. Semi-structured data is organized in a way that does not fit neatly into rows and columns but still has identifiable patterns or tags. Examples include sensor outputs formatted in JSON, XML files detailing asset specifications and tagged photos from facility inspections.
Semi-structured data combines the flexibility of unstructured data with the accessibility of structured data. For instance, a JSON file from a BMS sensor might include energy readings with metadata specifying time and location. FMs can analyze this data to identify trends without requiring extensive data cleaning, as would be the case with unstructured text.
Understanding and leveraging semi-structured data ensures FM professionals can harness insights from diverse sources, enriching their decision-making capabilities.
Types of data in FM
FM data comes in many different types and formats, each serving unique purposes. While structured and unstructured data categorize how information is organized, understanding data formats provides additional insight into how data is used in practice. In FM, these formats often include:
-
Text, such as maintenance logs, asset inventories, and budget reports. These are often structured but may also contain unstructured elements, such as freeform notes in work orders.
-
Audio, such as recordings, such as tenant complaints or inspection notes captured via mobile devices. Audio data, while unstructured, can be transcribed and analyzed to identify recurring issues or trends.
-
Visual includes images, videos, and blueprints and plays a critical role in FM. For example, photos from building inspections provide a rich source of information about asset conditions, while videos from CCTV systems enhance security.
Deciding what to collect
One common challenge in data initiatives is the temptation to collect all available data. This approach can be both costly and impractical, leading to overwhelming volumes of unused or irrelevant data. A more strategic approach involves identifying specific goals and determining how the data will be used to support decision-making. For example, if the goal is to optimize energy efficiency, focus on collecting data from systems such as HVAC performance, occupancy sensors and energy meters. By aligning data collection efforts with operational objectives, FMs can minimize costs, streamline analysis and ensure that the data gathered delivers actionable insights.
To put this into context, structured data might be the detailed work order history for an HVAC system, including dates, tasks performed and costs. Unstructured data, on the other hand, could be an email from a technician describing an unusual noise heard during an inspection. Both types of data are valuable, but they require different methods to extract and use the information effectively.
Data life cycle
Every piece of data goes through a life cycle, from collection to usage, much like a physical asset has its journey from purchase to maintenance to disposal. In FM, the data life cycle starts with collection, perhaps through sensors, manual entries or imported from external systems. It then moves to storage, processing and eventually analysis, where it becomes useful. Understanding where a piece of data is in its life cycle helps facility managers determine how reliable and actionable it is.
For instance, data collected in real time from occupancy sensors might be immediately useful for adjusting heating and cooling to save energy. Meanwhile, historical data on maintenance schedules might need to be processed and analyzed before it can inform long-term capital planning. Recognizing the different stages of data — from collection and storage to analysis and eventual archiving — ensures that FM professionals can derive maximum value from their data.
Core data literacy skills & principles
Data awareness
Imagine a building filled with untapped potential, like a historic structure with rooms yet to be discovered. Data awareness is about recognizing the available data sources and their potential to improve decision making. For FM professionals, data awareness means understanding what systems are generating data — be it building management systems (BMS), computerized maintenance management systems (CMMS) or even occupancy sensors — and realizing that this data can unlock new ways to optimize operations.
In practical terms, data awareness might involve recognizing that the building's lighting system generates data on energy usage patterns. This data, when properly analyzed, could reveal opportunities to reduce costs through demand-based lighting strategies. By being aware of all the available data sources, FM professionals can identify areas of improvement that might otherwise remain hidden.
Understanding data formats & types
Data formats are akin to different currencies. One type of data may be in the form of a spreadsheet (structured and ready for analysis), while another could be in PDF maintenance reports (unstructured and needing more work to be useful). FMs should understand how to “convert” these currencies — for example, extracting meaningful information from unstructured data sources — so they can make informed decisions regardless of how the information first appears.
Take the example of a PDF maintenance report. While it may contain rich insights about the condition of specific assets, it might not be immediately usable for analysis. FMs can use tools like optical character recognition (OCR) software to convert these reports into structured data that can be analyzed, such as entering information into a CMMS to help track performance trends over time.
Interpreting data accurately
Interpreting data is like reading an electrical schematic. The symbols and lines may look confusing without context, but when understood, they tell a story of connectivity and flow. In data terms, interpreting means ensuring that the numbers and observations are put in the correct context. If occupancy sensor data shows a room is empty 80 percent of the time, does that mean it is underutilized or is it reserved for high-priority events? Context matters, and a good data-literate professional always asks these kinds of questions.
For example, imagine analyzing work order completion times. If a trend shows that it takes longer to complete work orders during certain months, it might indicate a seasonal surge in workload or possibly reduced staffing during holiday periods. Without the proper context, an FM might incorrectly conclude that technicians are underperforming, when in fact there are external factors at play.
Data-driven decision making
Just as FM relies on maintenance checklists for consistent facility care, data-driven decision making relies on consistently interpreting data insights to guide actions. If energy use data indicates increased consumption, a data-literate FM professional does not just recognize the increase; they use the data to identify why it is happening and what actions could optimize usage — whether it is equipment maintenance, occupancy changes or altered setpoints.
Future planning is another critical area where data analysis excels. FMs use historical and real-time data to forecast trends and inform strategic decisions. For instance, analyzing occupancy trends can guide space allocation planning, while maintenance history can inform capital planning for asset replacements. By leveraging data for future planning, FMs can align operational strategies with long-term organizational goals.
Basic data terminology every FM professional should know
-
Data source: The origin of the data. In FM, this could be a sensor, a BMS or a spreadsheet with utility bills.
-
Metadata: Think of metadata as a label on a file folder; it tells what is inside without needing to open it. Metadata characterizes the data, providing details such as who created it, when it was created and how it is formatted, making it easier to search for, understand and connect with other data. For example, in an asset inventory record, metadata might include the asset's unique identifier, purchase date, maintenance schedule, manufacturer details and even its current status. This metadata allows FMs to easily locate specific assets, correlate them with maintenance records and understand their life cycle status — all of which contributes to more informed decision making.
-
Data Integrity: Just as structural integrity ensures a building remains safe, data integrity ensures the reliability and accuracy of data. Ensuring data is not corrupted or lost helps FM teams make confident decisions. For example, ensuring that sensor data is accurate by regularly calibrating devices is crucial for maintaining data integrity.
-
Data Quality: High-quality data is like clean water: essential for the “life” of decision making. Attributes of data quality include accuracy, completeness, consistency, timeliness and validity. If work order logs are incomplete, any analysis performed will also be incomplete, much like a blueprint missing key rooms or elements. FMs should periodically audit data to ensure it remains high quality, just as they would conduct a regular inspection of building systems.
-
Data features: Transparency is a key feature of data that directly supports FM. Transparency ensures that stakeholders can trace how data was collected, what tools were used and from where it was sourced. For instance, if energy consumption data is being analyzed, knowing whether it was derived from smart meters or manual readings is crucial for assessing its reliability. Transparent data practices also build trust, enabling FMs to confidently present insights and decisions to stakeholders. By maintaining transparency, organizations can ensure that their data processes remain credible and aligned with industry standards.
-
Data visualization: Tools like graphs, charts and dashboards that help translate complex data quickly. Imagine trying to explain energy usage trends with numbers alone versus showing a simple bar chart; visualization makes the complex intuitive. A good data visualization highlights key insights in a way that all stakeholders can understand, from technicians to executives.
Practical steps to enhance data literacy skills
Identify data sources
The first step toward becoming more data literate is to identify data sources with available access. Think of it as surveying a storeroom before starting a new project. Is data coming from energy meters, HVAC systems or perhaps historical work orders in a CMMS? Taking stock of what is available is essential.
For example, if an FM is trying to reduce water consumption, they should first identify the data sources that track water usage. This might include smart meters, historical utility bills and even data from irrigation control systems. By identifying and consolidating these data sources, they can begin to understand usage patterns and identify potential inefficiencies.
Ask the right questions
A data-literate facility manager knows to ask questions like: "Where did this data come from? Is it complete? How old is it?" Imagine trying to maintain equipment without knowing its maintenance history; working with data requires similar scrutiny. By asking these questions, one can evaluate the reliability and relevance of the data for specific FM decisions.
Asking questions also means challenging assumptions. For instance, if occupancy data suggests a particular area of a building is underutilized, the FM might ask whether this data aligns with actual usage patterns observed by staff. This kind of questioning helps ensure that decisions are based on accurate and complete information.
Basic data analysis techniques
FMs do not need to be statisticians to start using data analysis techniques. For example, trend analysis is like monitoring the temperature in a building across seasons to see patterns. An FM professional could look at energy use patterns over the past year to predict potential issues during peak usage periods.
Another basic technique is comparative analysis, which involves comparing current data against benchmarks or historical data. For instance, comparing current utility costs against the previous year’s figures can help identify anomalies or areas where efficiency measures are yielding results.
Simple tools like spreadsheets or CMMS reports can be used to conduct these types of analyses, making them accessible even for those without extensive data analysis training.
Effective data communication for FM professionals
Importance of clear communication
Good data communication is like writing a building inspection report — it needs to be clear and accessible to stakeholders with different levels of technical knowledge. When sharing data findings, use analogies and clear summaries. For example, instead of stating "energy use increased by 10 percent," explain that "this increase is equivalent to running five additional air conditioning units full-time during July."
Clear communication also means tailoring the message to the audience. When presenting to executives, focus on how data-driven actions impact the bottom line or improve tenant satisfaction. For technicians, focus on actionable insights, such as maintenance schedules or system adjustments that need to be made.
Visualizing data
A well-designed visualization is like a well-marked evacuation route: it guides the audience clearly to the information they need. Charts and graphs can often tell the story of data more effectively than numbers alone. A line chart showing equipment performance over time can quickly highlight when an anomaly occurred, making it easy for decision makers to pinpoint where action may be needed.
Insert Dashboard mockup
Consider using dashboards to present multiple data points in an easily digestible format. A dashboard could include real-time energy usage, current maintenance requests and occupancy levels all in one place. This type of visualization provides a comprehensive view at a glance, helping FM professionals prioritize actions and monitor progress.
Additional resources for building data literacy
-
Online courses:
-
Free tools:
-
Google Sheets for practicing data manipulation and visualization.
-
Microsoft Power BI (free version) for developing data dashboards.
-
-
Reading:
-
The Data Literacy Project provides an excellent starting point for understanding core concepts: The Data Literacy Project.
-
Next steps
Data literacy is not just about crunching numbers; it is about making more informed decisions that benefit facilities, occupants and bottom line. By learning the language of data, FM professionals can transform how buildings are managed, taking advantage of the wealth of information available to enhance operational performance and sustainability.
Click here to read Part II: Validation, quality & management for reliable decisions
Click here to read Part III: Governance frameworks
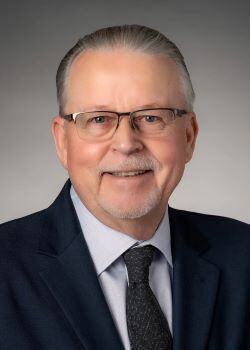
Read more on Technology and Emerging Topics
Explore All FMJ Topics