Data Unlocked:
Part II: Validation, quality & management for reliable decisions
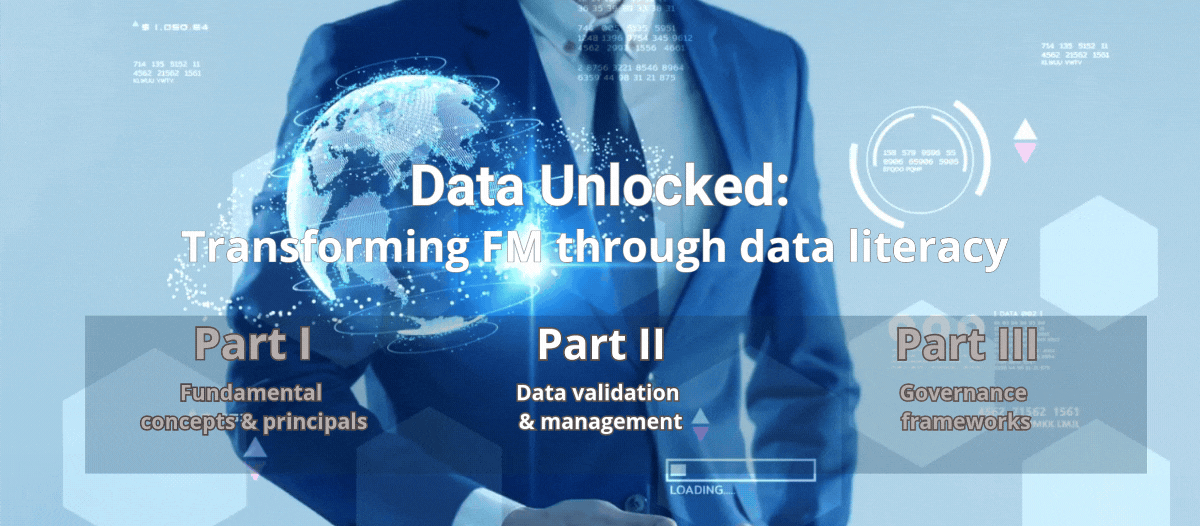
Editor’s note: This is the second article in a three-part series discussing the fundamental concepts of data literacy, validation and governance and FM.
Imagine data as the core infrastructure that supports an entire building management operation. Without a solid foundation of high-quality information, even the most advanced facility management strategies will falter. FMs must ensure their data is accurate, reliable and consistent. Maintaining a solid data foundation will support effective decision-making, improve operational efficiency and enhance the value of facility management initiatives.
Understanding data quality in FM
Defining data quality: What makes data fit for use
Data quality in FM is like selecting the right components for a critical system. Imagine managing HVAC equipment and deciding between using substandard or high-quality parts. Naturally, choosing high-quality components ensures performance and reliability. The same principle applies to data; it must be dependable to support sound decisions. High-quality data stands out through five essential attributes: accuracy, completeness, consistency, timeliness and validity.
-
Accuracy ensures that information reflects reality, much like how precise technical specifications for building systems prevent operational errors. For instance, inaccurate records about HVAC equipment could lead to improper maintenance or costly inefficiencies.
-
Completeness means having all the necessary details to make informed decisions. Imagine assessing utility performance without full submeter data. Gaps in information leave decision-makers struggling to see the full picture, just as missing parts in a blueprint can stall a construction project.
-
Consistency aligns data across systems and records, ensuring that everyone works with the same understanding. When asset names vary between procurement and maintenance systems, it creates confusion and inefficiencies. Consistent data ensures smooth operations, akin to standardized procedures on a construction site.
-
Timeliness guarantees that data is current and actionable. Outdated information—like relying on an old service contract for compliance audits—can lead to errors and regulatory violations. Timely data reflects present conditions, enabling swift and effective responses.
-
Validity ensures that data values are realistic and contextually appropriate. Consider HVAC system performance metrics: if recorded values do not align with real-time conditions, decisions based on that data will be flawed. Valid data allows facility managers to make decisions that mirror operational realities.
By understanding and prioritizing these attributes, FMs can ensure that their data is both fit for use and a powerful tool for driving operational excellence.
The role of data quality in effective decision-making
Imagine attempting to adjust the energy profile of a building based on unreliable data—it would lead to inefficiencies and unnecessary expenses. High-quality data provides the confidence needed to make informed decisions, whether it is planning preventive maintenance, managing budgets, or optimizing energy consumption. Without reliable information, FMs run the risk of increased costs, missed opportunities, and potential operational setbacks.
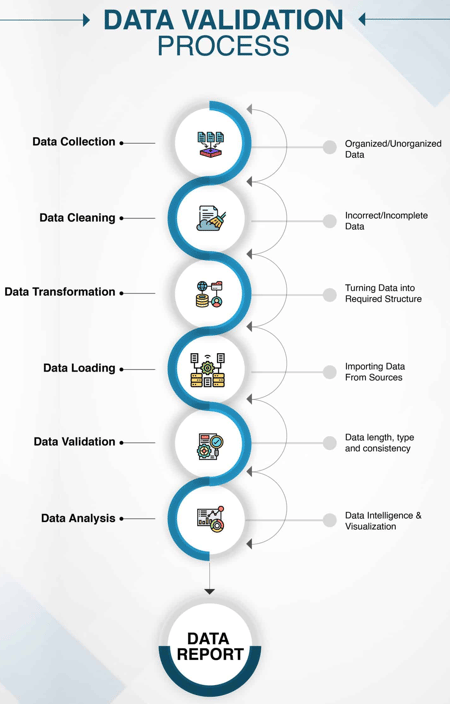
Data validation: Ensuring data accuracy from the start
What is data validation?
Data validation can be compared to conducting a quality assessment before deploying a new building automation system (BAS). Just as verifying the accuracy and compatibility of the control modules, validation ensures that data entering systems meets defined standards, preventing inaccuracies that might impact operations. For FMs, this means confirming that information about assets, maintenance schedules or energy usage is accurate from the beginning.
Types of data validation
-
Syntax validation: This is like ensuring that all contract documents are formatted correctly before submission. Syntax validation checks that data follows a predefined structure. For example, verifying that dates are recorded correctly as "DD-MM-YYYY" ensures consistency.
-
Content validation: Think of content validation as ensuring that the set points in your building automation system align with actual comfort levels. Content validation confirms that data values make sense in context. For instance, if a recorded space temperature is 500 F, it is clearly incorrect and requires correction.
-
Cross-validation techniques: Cross-validation is akin to ensuring that data from different systems within a building, like energy management and maintenance, align correctly. This technique compares data across systems to ensure consistency. For example, asset locations should match maintenance, procurement and inventory systems.
Methods & tools for data validation in FM systems
Data validation can be automated or manual—like automated systems checks and manual inspections in facility operations. Automated tools can be integrated into systems like a computerized maintenance management system (CMMS) that flags incorrect data formats or missing fields. Manual validation, akin to an experienced technician reviewing the work, helps catch issues that automation might miss, especially in qualitative areas. A balanced approach ensures comprehensive data accuracy.
Measuring data quality in FM
Key metrics for evaluating data quality
Measuring data quality requires specific metrics, much like evaluating building system performance.
-
Completeness: Completeness ensures no critical piece of information is missing. Imagine managing an asset inventory but lacking crucial details like an installation date or warranty period. For example, an FM might measure completeness by auditing asset records to ensure that at least 95 percent of all entries include mandatory fields such as installation dates, maintenance schedules, and warranty details. Missing data leaves gaps that hinder the decision-making process.
-
Accuracy: Accurate data is crucial for effective operations. For instance, inaccurate records regarding HVAC service frequency might lead to failures, impacting tenant satisfaction. Accuracy can be measured by comparing recorded data against source documents or field inspections; for example, verifying that 98 percent of HVAC maintenance logs match service technician reports ensures reliable records. Just like proper calibration ensures equipment operates efficiently, accuracy ensures that data drives reliable actions.
-
Consistency: Consistent data ensures all stakeholders have the same understanding, like aligning procurement records with maintenance needs. If the maintenance system and procurement system have different asset IDs for the same equipment, it could lead to miscommunication and delays. Measuring consistency might involve reviewing cross-system data alignment, such as ensuring that 100 percent of asset IDs in the procurement database match those in the CMMS.
-
Timeliness: Timely data allows FMs to make decisions that reflect current conditions. An FM can measure timeliness by tracking the percentage of updates made within a predefined time frame—such as ensuring that 95 percent of work orders are updated in the system within 24 hours of completion.
Tools & techniques for measuring data quality
-
Data profiling tools: These tools analyze datasets to determine their completeness and integrity, akin to assessing building condition through a facility condition index.
-
Quality dashboards: Quality dashboards provide a visual overview of data health, like an energy management dashboard that monitors building energy performance.
-
Scorecards and data quality indicators: Scorecards assess data reliability, much like performance metrics for systems. A high score indicates the data is robust enough to base significant operational decisions on.
Common challenges & solutions
Maintaining data quality in FFM presents challenges like optimizing a complex building system.
-
Incomplete data: Incomplete data is a frequent issue, much like an HVAC system missing a key component. Regular audits help identify and address these gaps to prevent disruptions.
-
Inconsistencies across systems: Inconsistencies in data can arise from two primary issues: inconsistencies across systems and inconsistencies within a single system.
-
Cross-system inconsistencies: These occur when data across different systems, such as procurement, maintenance and inventory, do not align.
-
Within-system inconsistencies: These happen when data is not collected or entered consistently within the same system.
-
Addressing these inconsistencies requires establishing robust data governance policies, standardizing data entry protocols, and employing tools that enable synchronization across platforms. By tackling both types of inconsistencies, facility managers can ensure smoother operations and more accurate decision-making.
-
Data Maintenance: Maintaining data over time is essential for ensuring its continued accuracy, relevance, and usability. Data maintenance involves regular updates, audits, and corrections to address inconsistencies, outdated information, or gaps. For instance, asset inventory data must be periodically reviewed to account for replacements, repairs, or decommissioned equipment. Neglecting data maintenance can lead to data credibility concerns, such as using inaccurate maintenance schedules or failing to track asset performance trends. By establishing consistent maintenance practices, facility managers can ensure their data remains a reliable foundation for decision-making.
-
Data duplication: Duplicate data entries lead to conflicting records, similar to having mismatched sensors for the same HVAC unit. Master data management (MDM) practices help maintain a unified source of truth.
MDM in FM
MDM explained
MDM in FM is analogous to having a comprehensive building information model (BIM) that serves as the authoritative source of all asset information. In FM, MDM means creating a single, unified repository for all critical data, including asset specifications, vendor details, and maintenance schedules. This central source ensures consistency and reliability in operational decisions.
Implementing MDM: Best practices
-
Identifying critical data sets for FM: Start by identifying essential data, such as asset inventories, service contracts, and maintenance history. Asset inventories should contain attributes like installation dates, service records, and warranty periods.
-
Establishing data standards and governance: Establishing data standards is like adhering to a standardized protocol for energy auditing. Classification systems such as Omniclass or UniFormat are useful in maintaining uniformity across various datasets.
-
Tools and techniques for MDM implementation: Implementing MDM often involves using tools like an enterprise resource planning ERP system that integrates data from procurement, maintenance and operational departments. Much like a building management system integrates HVAC, lighting and security, MDM centralizes critical information.
Practical examples of MDM in FM
Consider a large corporate campus implementing MDM to consolidate data across maintenance and operations. Standardizing data allowed maintenance teams and procurement managers to access consistent information, reducing downtime and improving efficiency. This approach is like using a centralized energy monitoring system that all stakeholders can access to optimize building performance.
Case Study: Real-world application of data quality in FM
Highlighting a facility that improved operations through enhanced data quality
The Georgia Institute of Technology faced challenges managing facilities data across its extensive campus. Relying on 36 different software systems led to issues with consistency and outdated data. By focusing on data validation and MDM, Georgia Tech significantly streamlined their systems, reduced maintenance response times, and improved overall operational efficiency. Their success highlights the practical benefits of focusing on data quality in FM.
Practical steps to improve data quality
Creating data quality guidelines
Creating data quality guidelines is like establishing standard operating procedures for building safety. These guidelines dictate how data should be collected, stored and updated. FMs can reference industry standards such as ISO 8000 or leverage best practices from professional associations such as IFMA to create effective data quality protocols.
Training FM staff on data practices
Training staff in data quality practices is crucial. It ensures all personnel are certified in energy management to guarantee effective operations. FMs should receive and provide data literacy training that emphasizes accurate data entry and updates. Platforms like Coursera or edX offer foundational courses, and IFMA offers credential training tailored to FM needs.
Regular data audits & quality checks
Data audits are like conducting facility condition assessments—they help ensure the accuracy and completeness of data. Routine audits allow FMs to identify discrepancies early, preventing larger issues from developing. Systematic quality checks maintain data accuracy, much like periodic recalibration ensures sensors provide correct readings.
Tools & resources for data quality improvement
Suggested software solutions & techniques
FMs can explore data profiling and cleaning tools to enhance data quality. Some helpful search terms for finding suitable tools include:
-
"Top data profiling tools for facilities management"
-
"Best commercial data cleaning software 2024"
-
"Data validation tools for asset management"
These searches will return a variety of available solutions that can be tailored to meet specific FM requirements.
Additional learning resources
Online platforms like Coursera and edX offer courses such as Data Literacy Fundamentals and Data Quality for Business Professionals, which can provide Facility Managers with the skills needed to better understand and maintain data quality. Additionally, organizations like the Data Literacy Project offer free resources for building foundational data skills.
Conclusion & next steps
High-quality data is the backbone of effective FM. Just as building systems depend on high-quality components for reliable operations, the quality of decision-making relies on accurate and consistent data. By focusing on data validation, quality metrics, and MDM, FMs can enhance operations, improve cost-effectiveness and support strategic objectives.
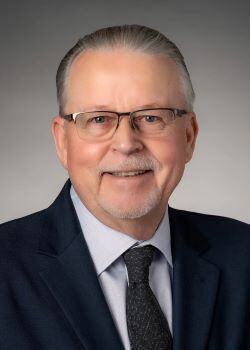
References
Data Literacy Fundamentals on Coursera - A beginner-friendly course on understanding and working with data. Available for free audit: coursera.org/learn/sas-data-literacy-essentials
Data Literacy Foundations on edX - A course offering foundational data literacy skills, provided by the Rochester Institute of Technology: edx.org/learn/data-analysis/rochester-institute-of-technology-data-literacy-foundations
The Data Literacy Project - A comprehensive resource for building data literacy skills: https://thedataliteracyproject.org/